Generative AI
Gen AI x Design Research
What If Generative AI Could Redefine Design Research?
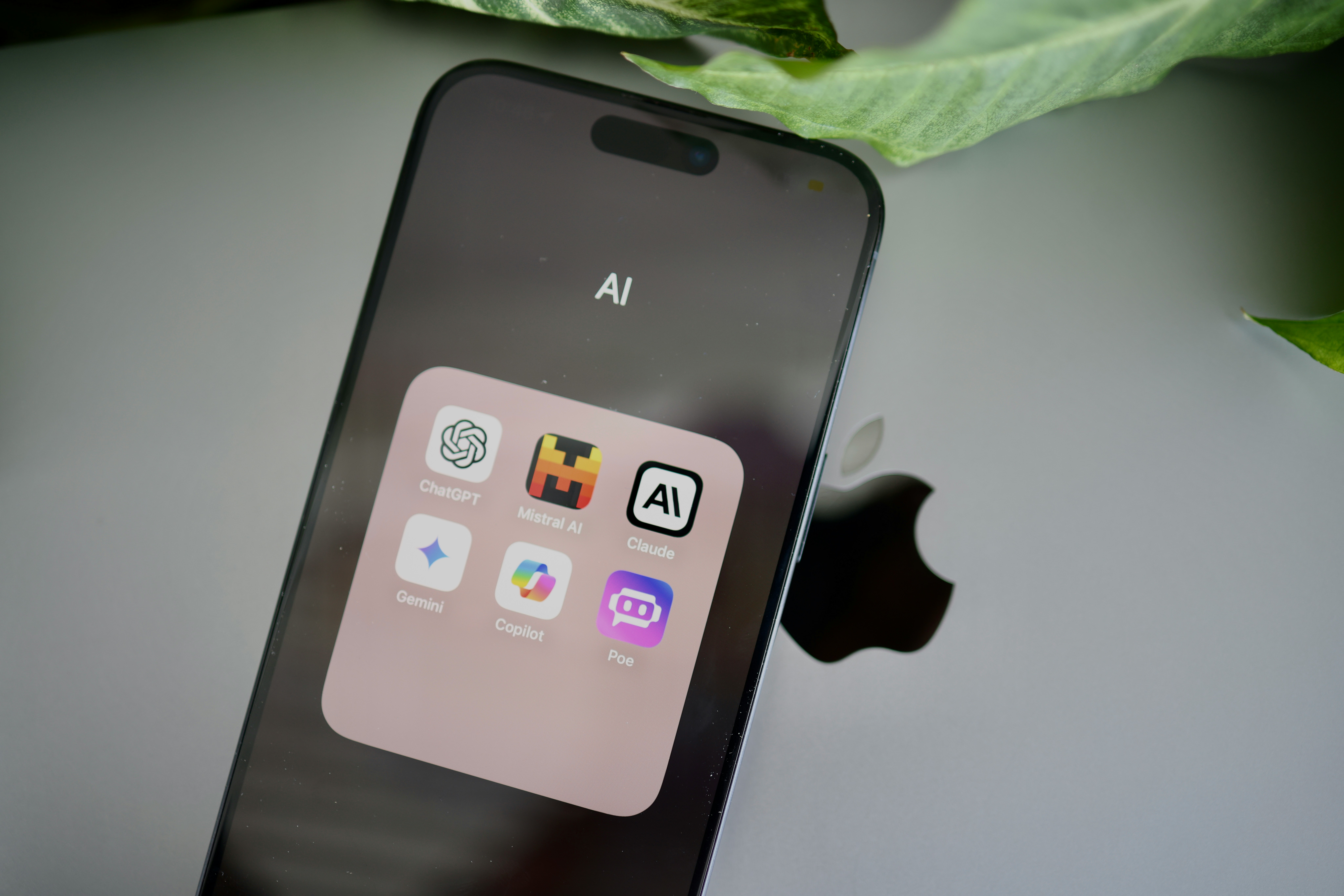
Team
Sejal Amrutkar
Ayushi Gupta
Role
UX Researcher
Tools
Miro, Canva, Qualtrics, MS Office, Adobe Illustrator
Timeline
5 months
Research Focus
Our research centered on understanding the transformative impact of Generative AI on design research, emphasizing the following:
Evolution of design tools and methodologies.
The influence of AI on research efficiency and workflows.
Risks of professional stagnation for practitioners unwilling to adopt AI advancements.
Outcome
The research led to the development of 3 key opportunity areas, which were validated by AI-driven design professionals. After gathering their feedback, two of the directions were merged to address the issue of bias in AI models—a recurring challenge we identified. This problem statement became our focus for further ideation and refinement.
Key Activities
Data Collection:
Desk Research
16 interviews
3 AI Events
2 Experiments Conducted
Data Analysis:
Competitive Landscape Audit
Archetypes
User Journey Mapping
Affinity and Signal Mapping
Thematic, Process, In-vivo Coding
PESTS Analysis
6E Framework
Rose-Thorn-Bud
Process
Opportunity Areas and Potential Directions
We identified three critical opportunity areas, each addressing unique facets of AI integration.
Collaboration and Integration
How might we create a human-AI collaborative ecosystem to optimize collaboration and productivity through a relationship-focused approach to AI integration?
Reliability and Authenticity
How might we build credibility within AI-driven research through transparent, verifiable, and authentic measures that empower stakeholders to make informed decisions?
Experimentation and Exploration
How might we foster an efficient exploration journey that helps individuals develop
a comfortable interaction model with AI while emphasizing the importance of contextual understanding for desired outcomes?
Desk Research Highlights
We analyzed scholarly articles, business journal reports, published studies, research papers, articles, tech and design surveys, and blogs/vlogs on relevant topics to frame our understanding of the current AI landscape in design research. Our findings emphasized the growing gap between early adopters and those resistant to change.
Repercussions for design professionals if they don’t adapt:
Limited Competitiveness
Reduced efficiency and resource utilization
Missed opportunities in data-driven Insights
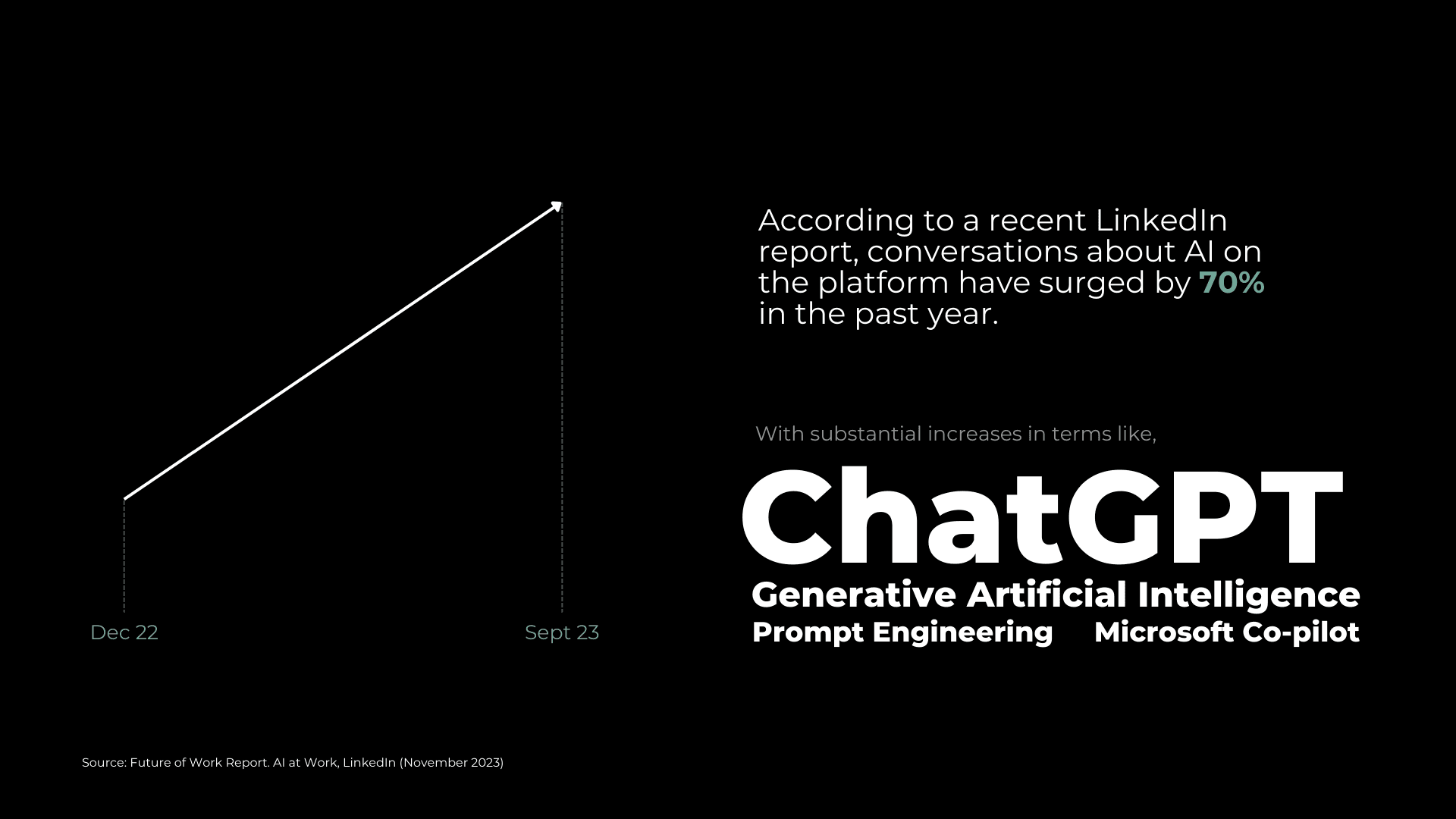
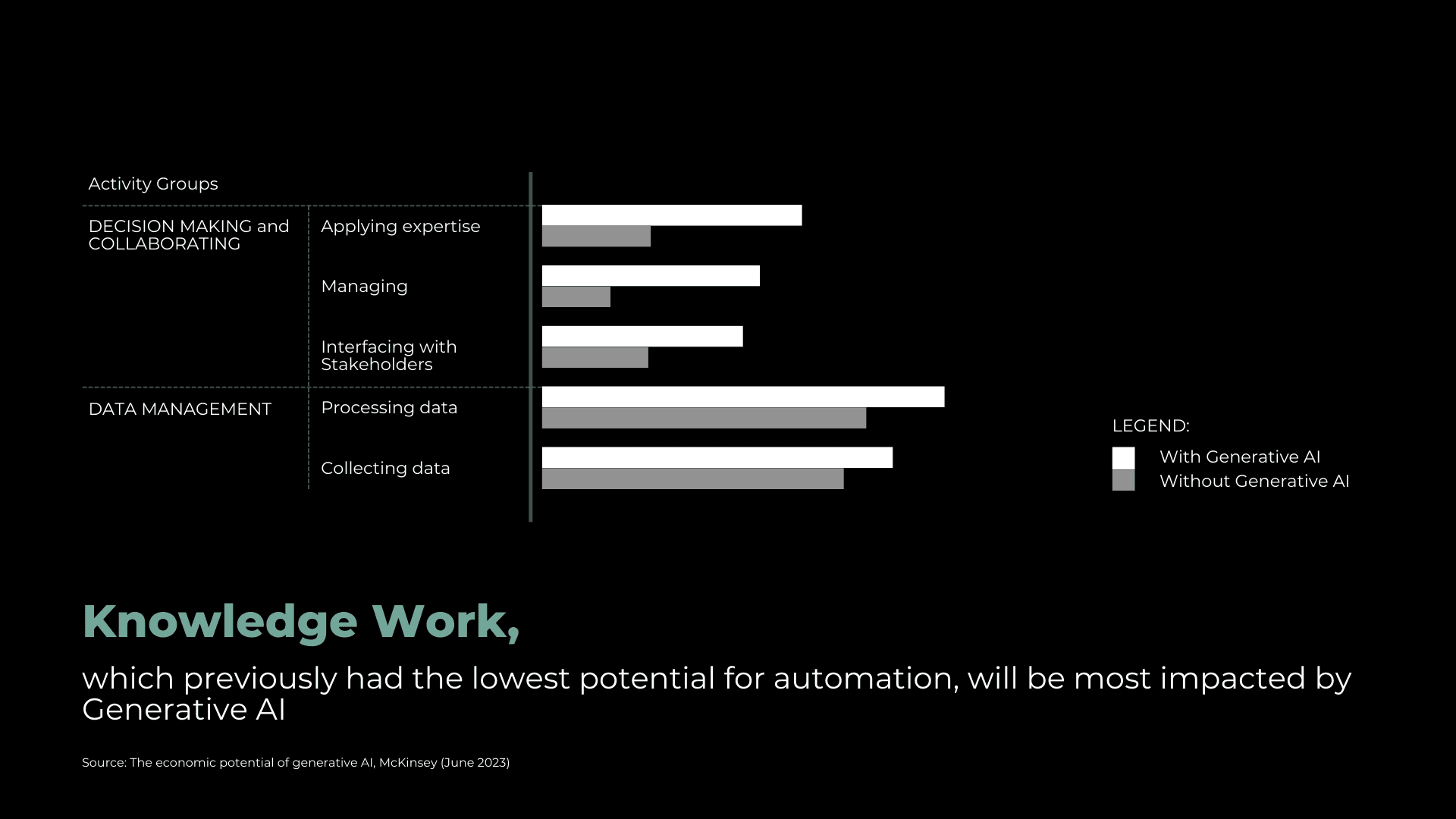
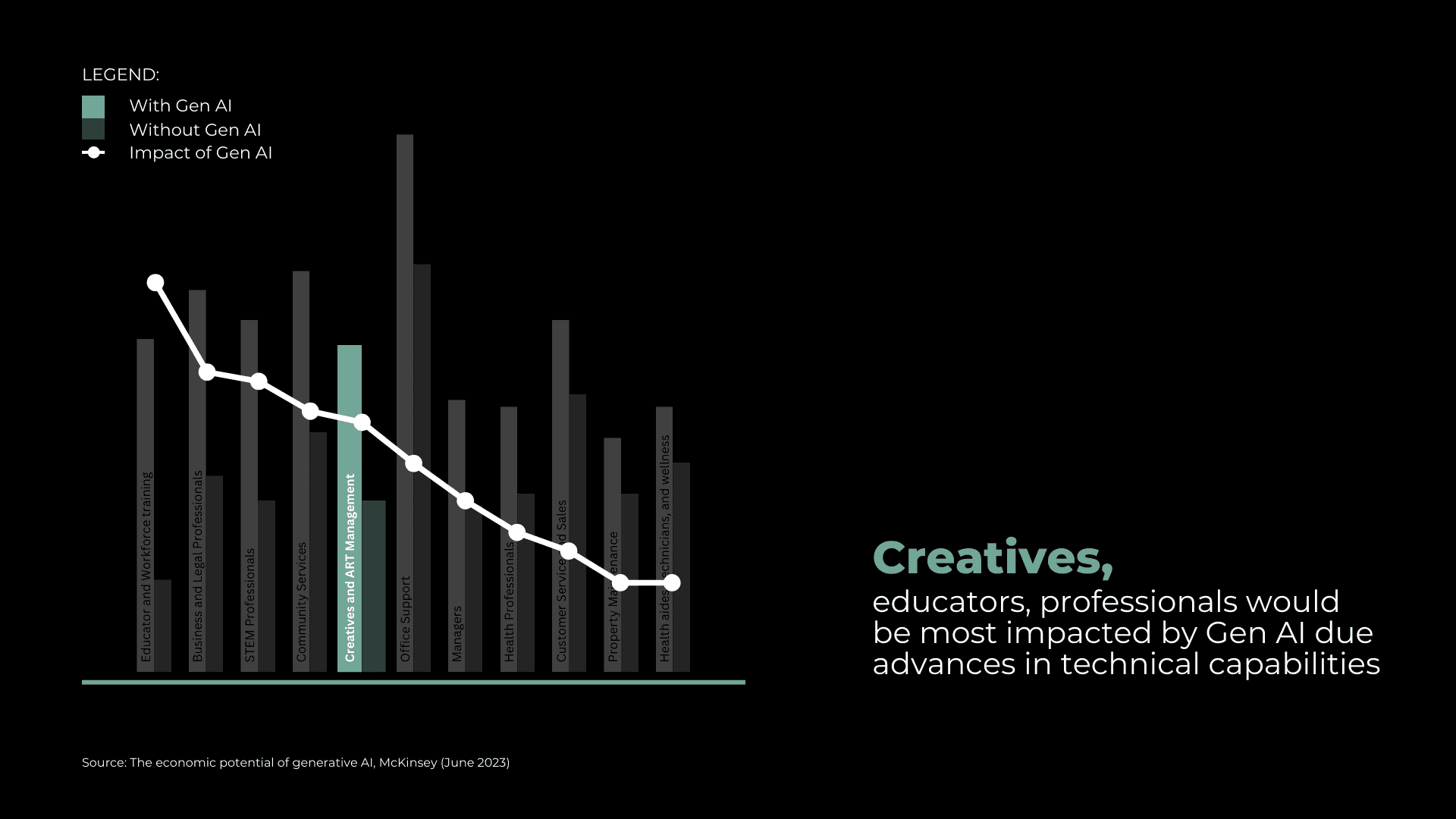
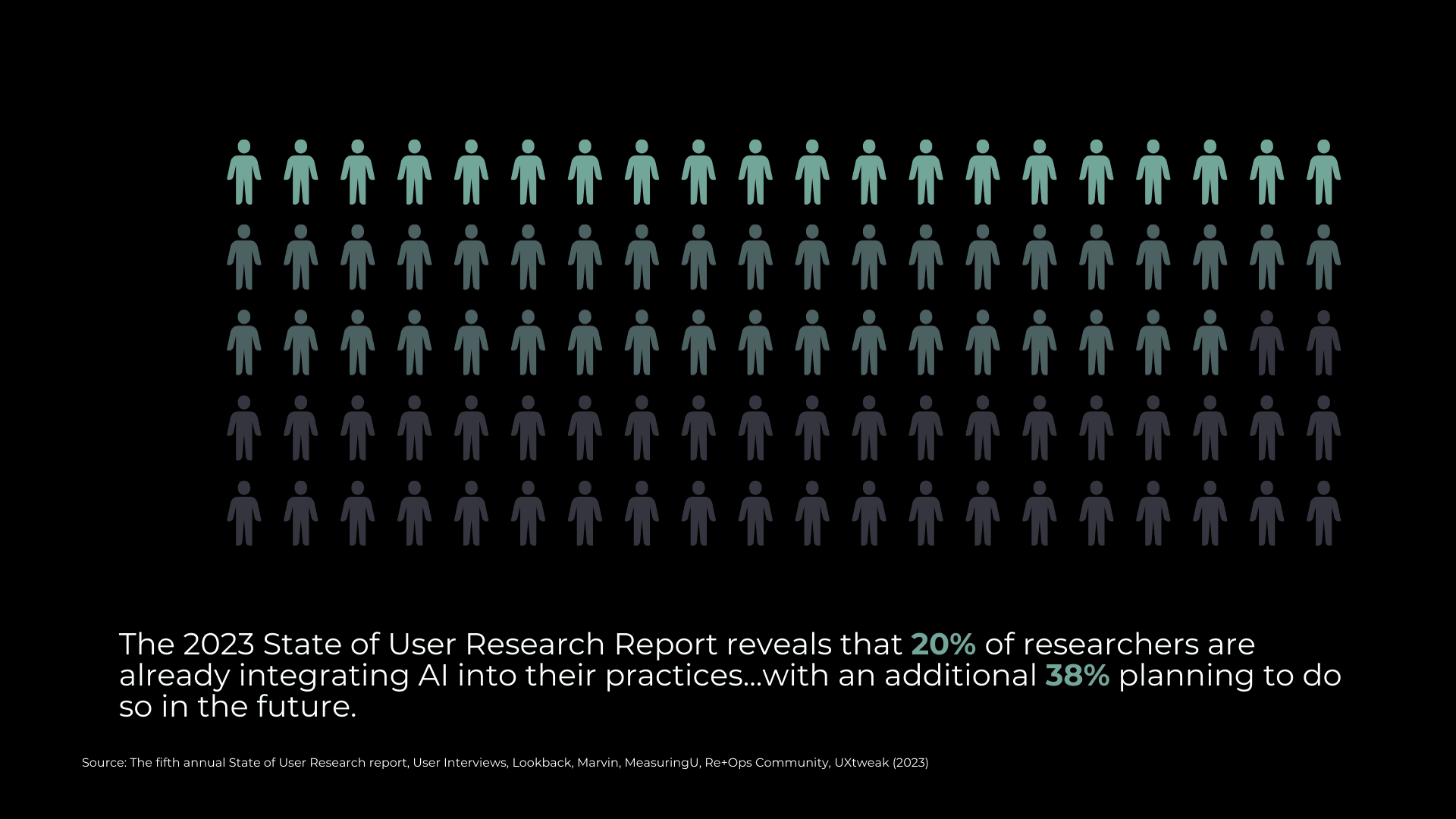
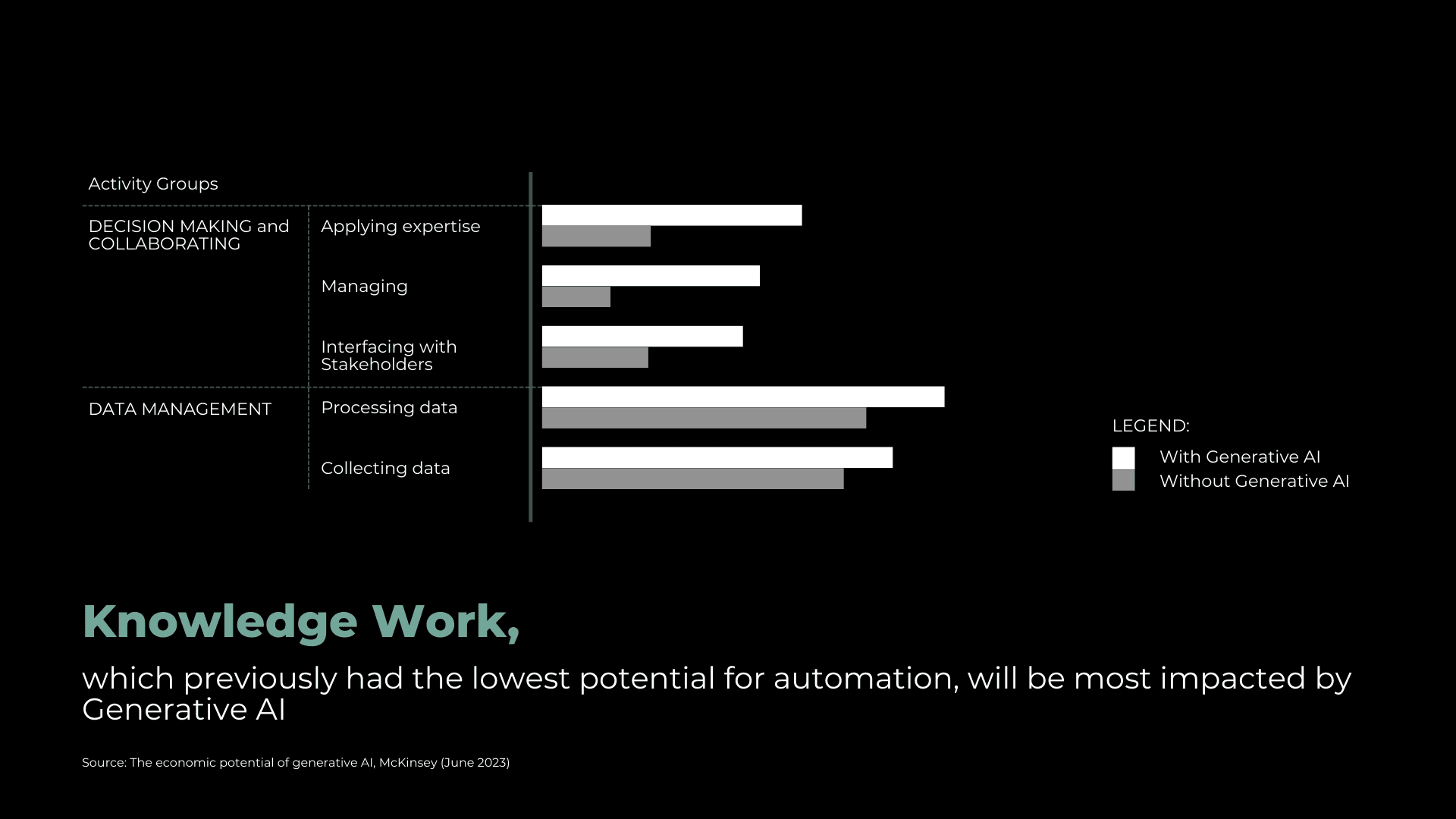
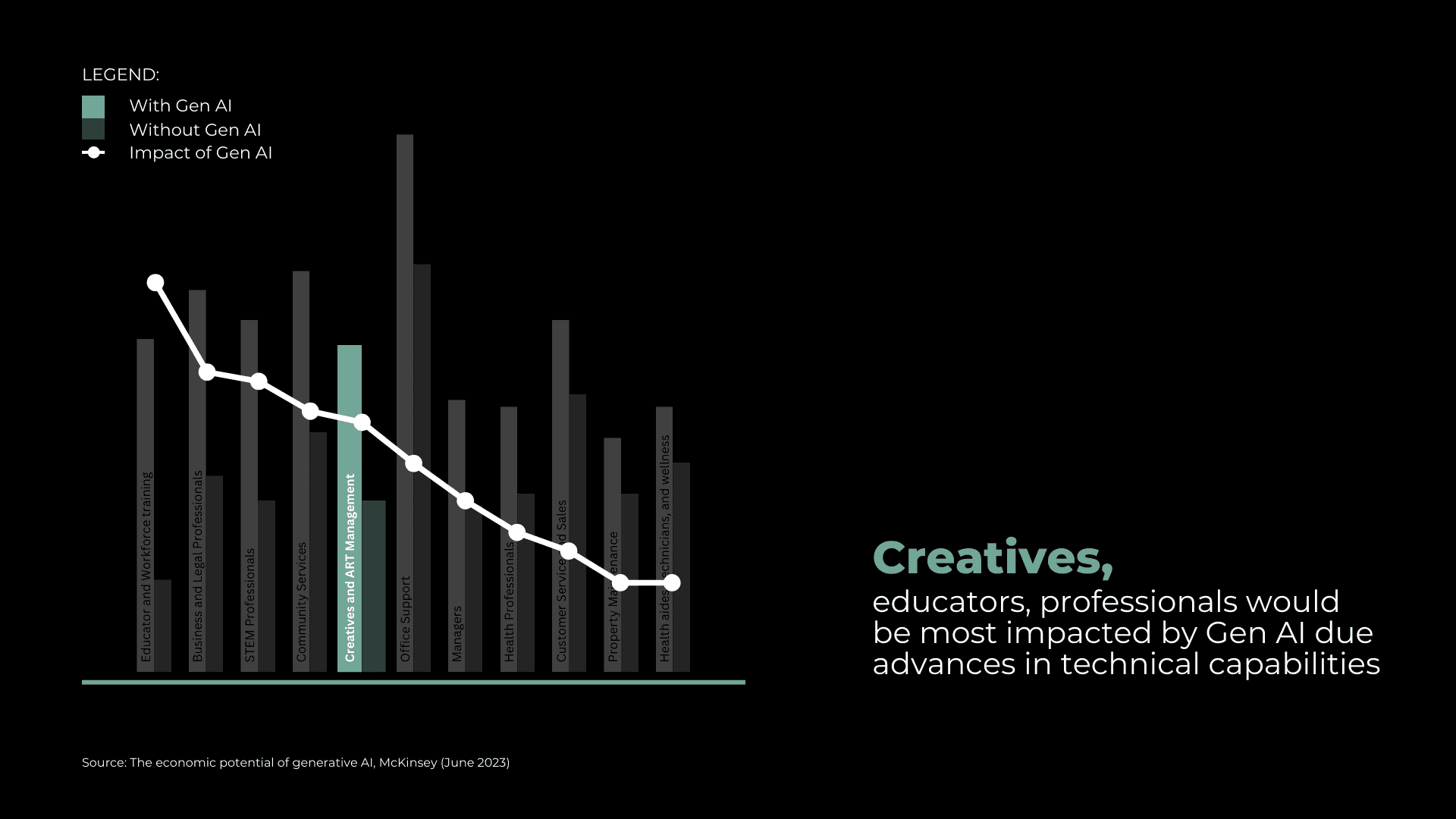
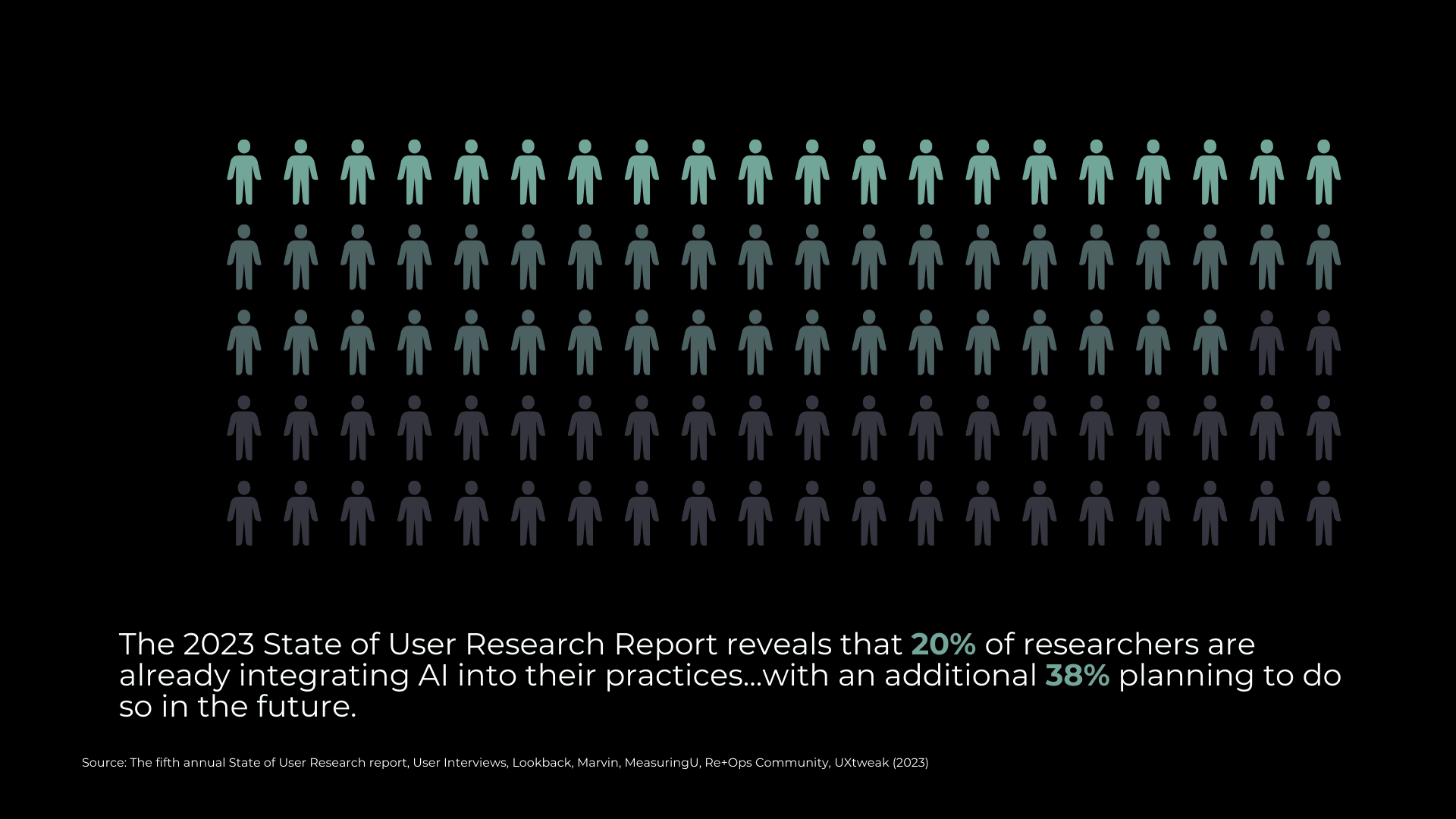
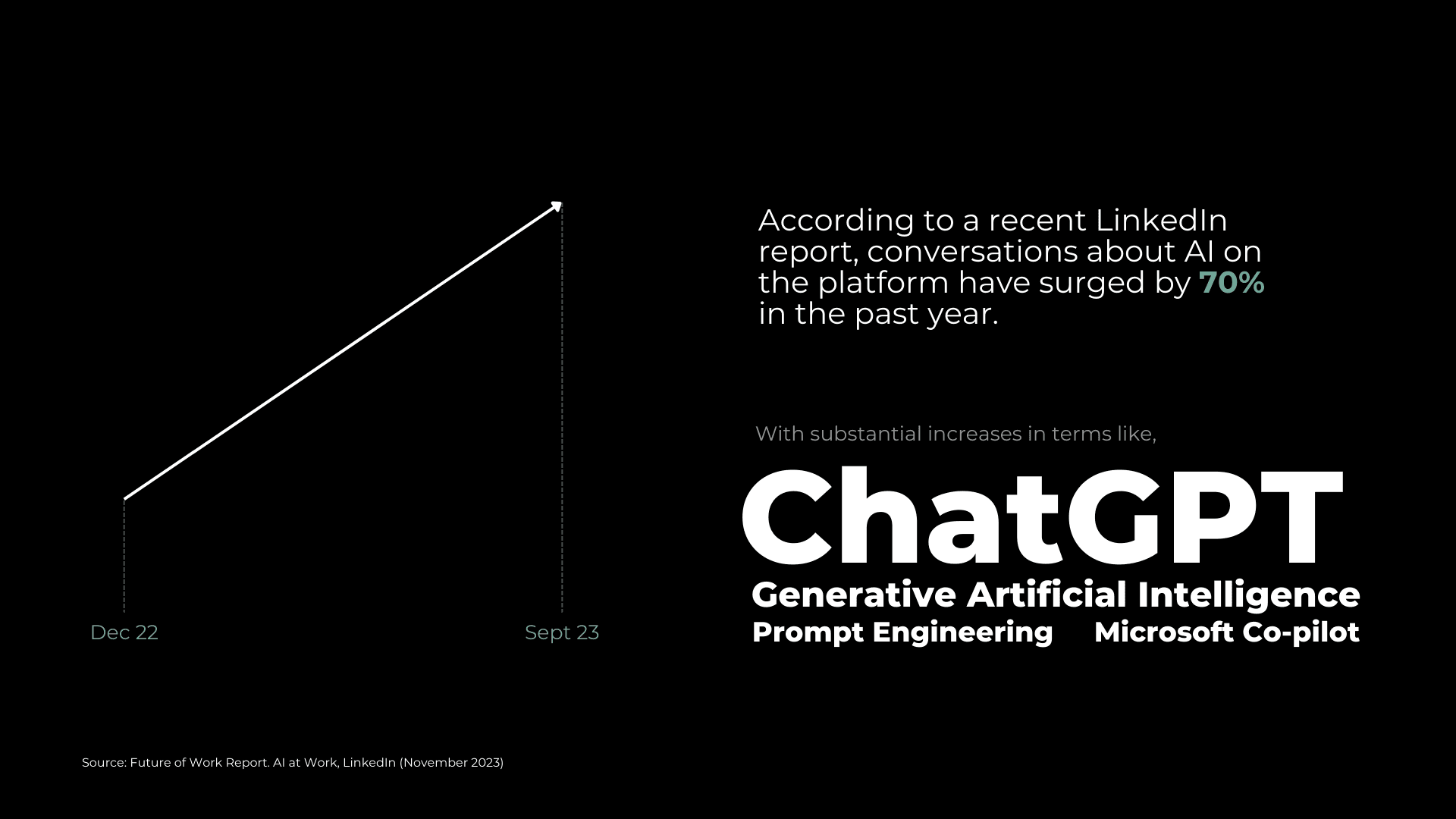
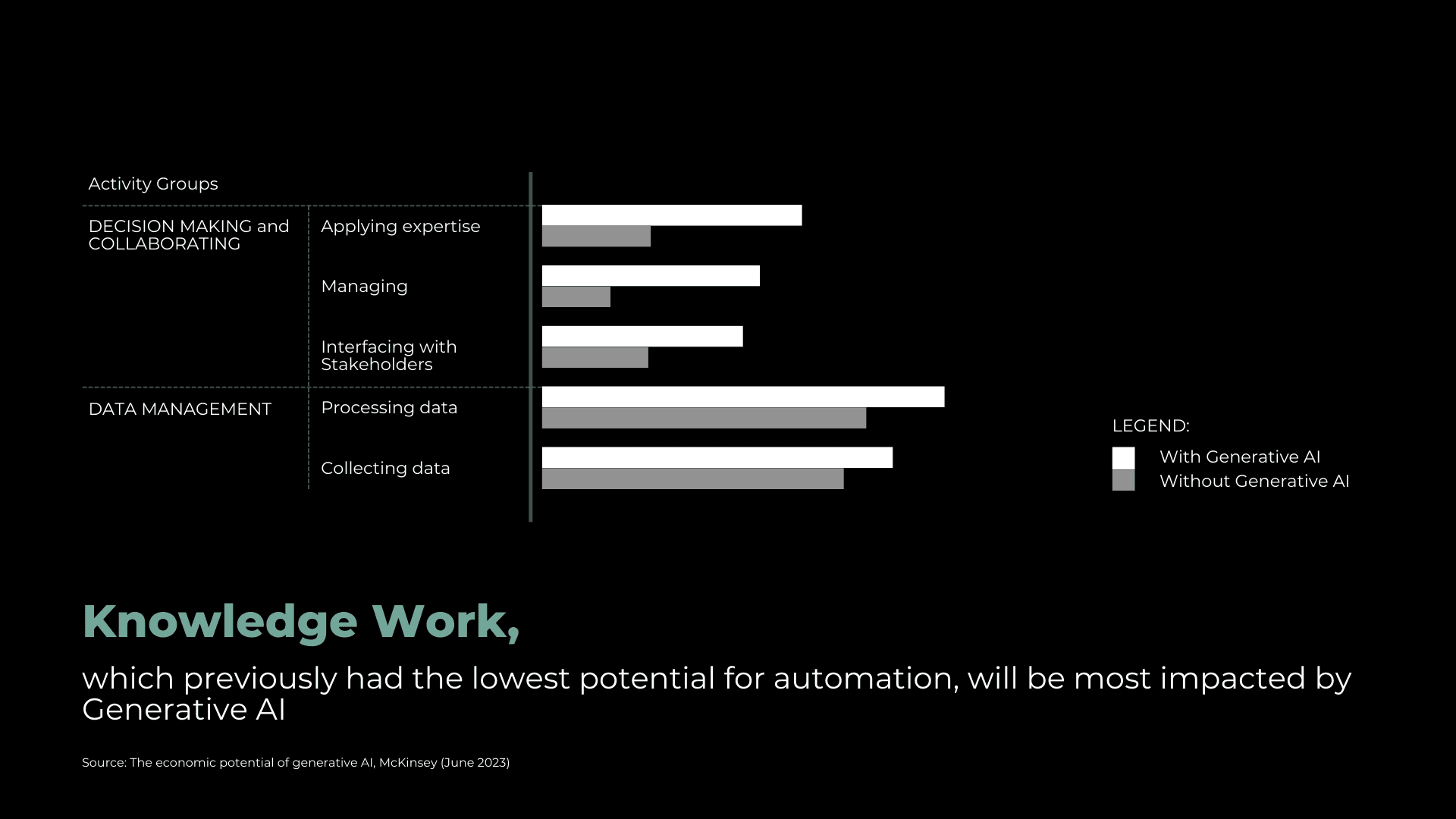
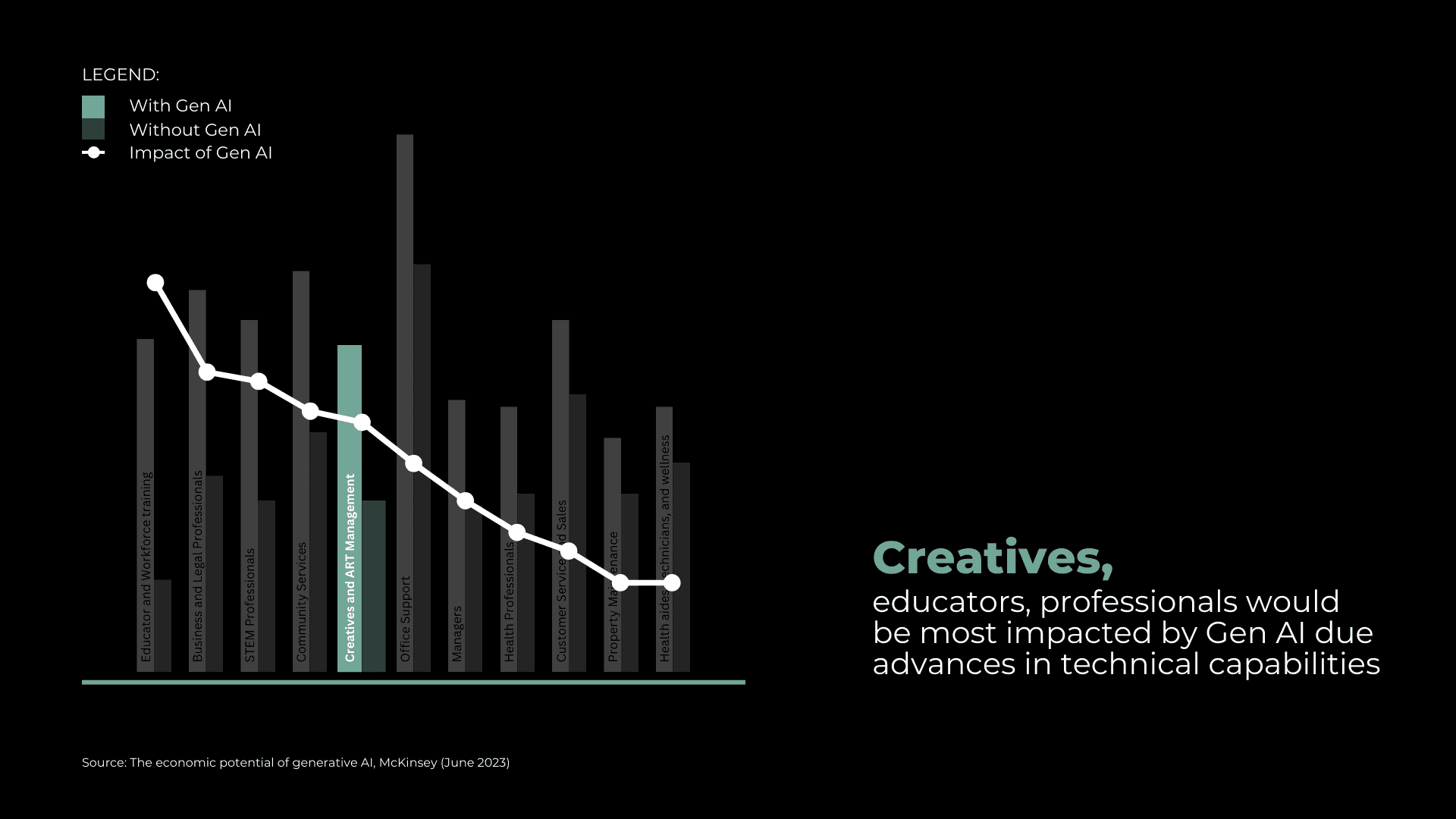
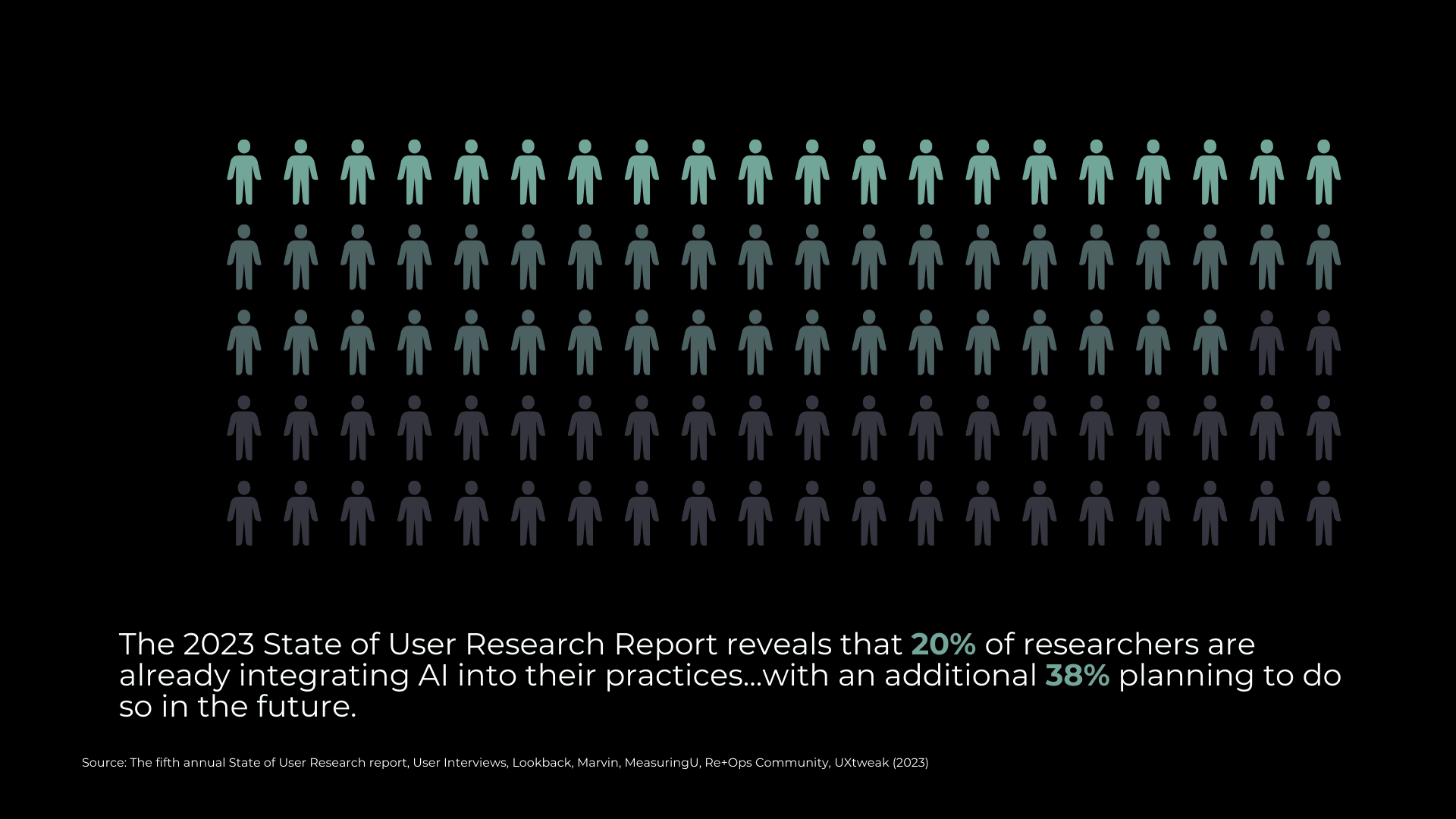
What Did the Users Have to Say?
Through discussions with student and professional design researchers, educators, and AI domain experts, we gained valuable insights into current perceptions of AI and its application in design practices.
Emerging Interaction Models
Users noted the growing role of AI as a co-pilot in design processes, enhancing creativity through collaborative approaches. By managing data-intensive tasks, AI allows human researchers to concentrate on strategic and conceptual work.
Resource Management in AI-Integrated Design
AI helps allocate time, finances, and personnel efficiently by automating routine data analysis. However, challenges such as information overload and trust issues regarding AI outputs persist.
Cultural Context and Bias Awareness
Grasping users' cultural and linguistic nuances is key to generating meaningful insights. Building trust through transparency helps tackle biases in AI models, aligning AI-generated findings with the authentic experiences of communities.
Dichotomy between Users
We discovered 2 main extremities of the user groups:
AI Enthusiasts - The enthusiasts were keen on exploring the potential of Gen AI, formulating and adjusting their collaborative learning experiences as they try to integrate it into their processes.
AI Skeptics - The skeptics showed major concerns regarding the threat to community experiences, specifically situating scopes outside the communities due to a loss in the quality of the community-designer relationship.
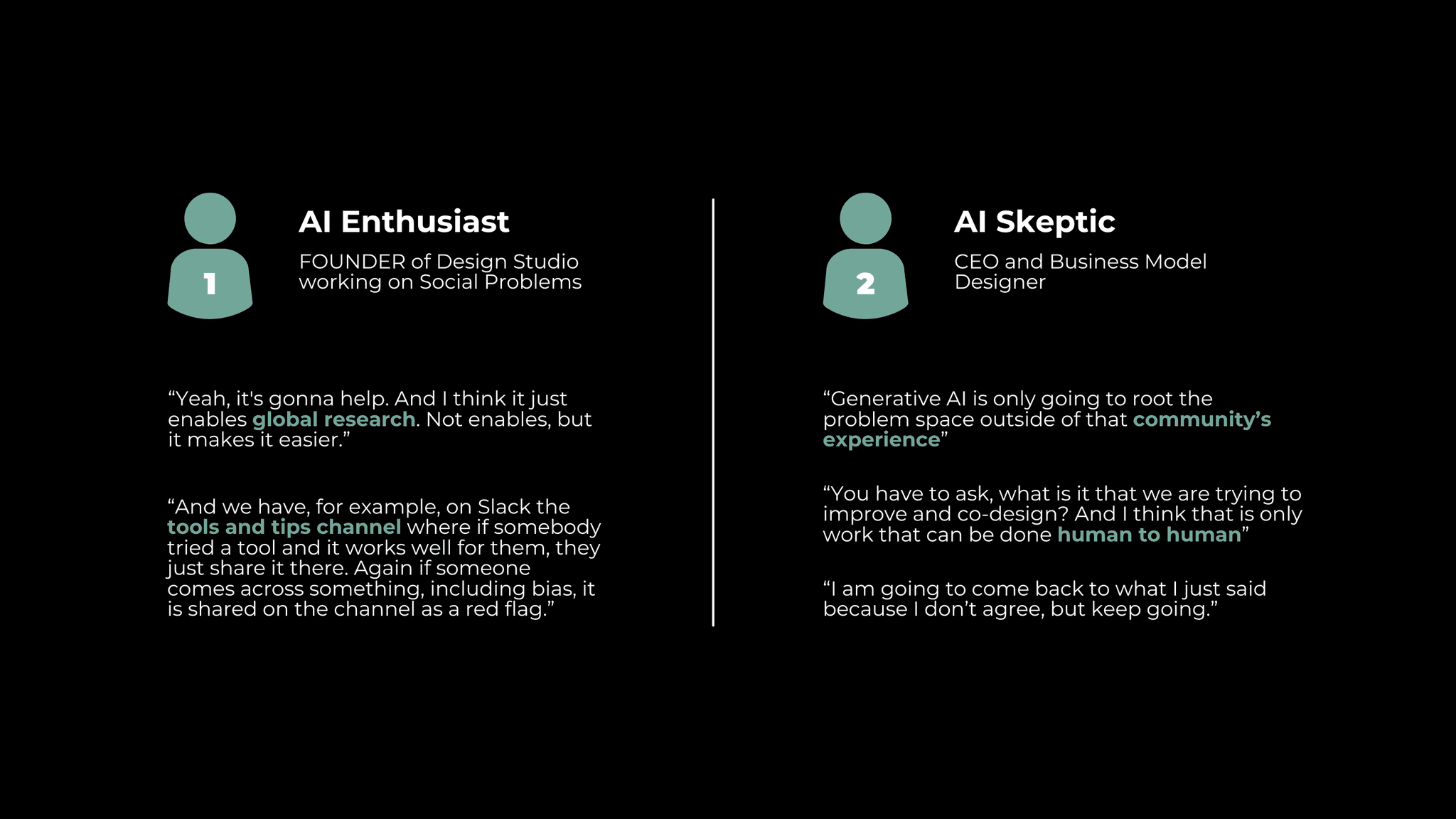
AI Experiment for Hypothesis Testing
As part of our research, we conducted a mini-experiment to compare the thematic analysis capabilities of a human researcher with those of an AI-assisted researcher. Ayushi and I alternated between using ChatGPT and Gemini to process our interview summaries and extract key themes from the data.
AI Tools: ChatGPT, Gemini
Output evaluation criteria: Quality, Time required to get the desired results, Number of prompt iterations
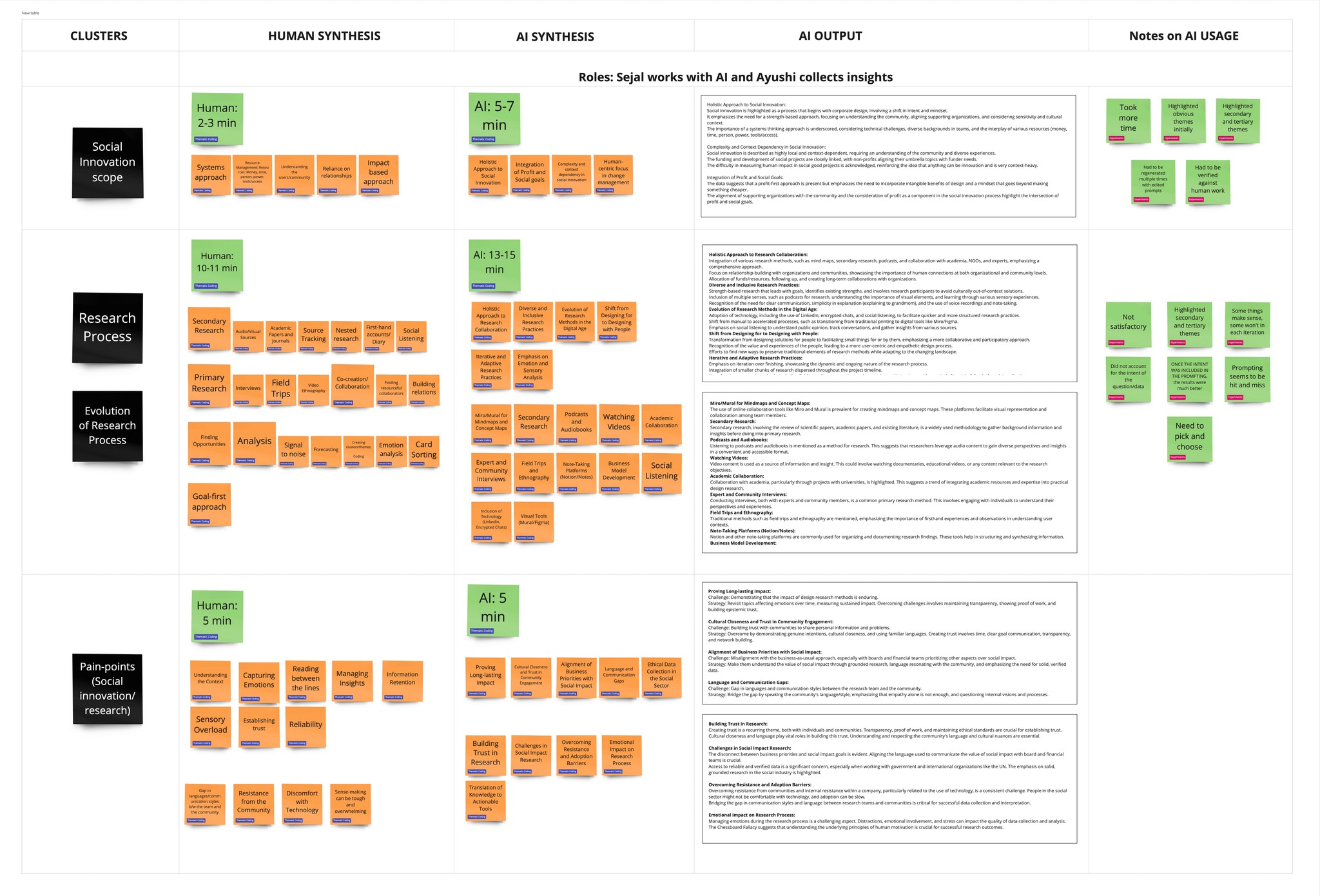
Trade-offs Made
The experiment was originally designed to be a 2-day study, with 3 sample groups exposed to different aspects of the AI process. However, due to logistical and time constraints, the participant recruitment was slowed down. Hence, we decided to do an internal experiment to study how AI would impact our research first-hand. We complemented this with a paper published in Sept 2023 that studied the Effects of AI on Knowledge Worker Productivity and Quality conducted with BCG.
Key Discoveries
Simplification vs. Hierarchy
While AI effectively simplified the data, it struggled to establish an appropriate hierarchy. It processed well-organized information efficiently and identified some "obvious" themes that we had overlooked.
Importance of Iteration
Achieving optimal responses required multiple iterations. Intent and context were critical in guiding the AI through follow-up questions and keyword searches to yield the desired results.
Need for Human Verification
Cross-verifying AI-generated insights with human analysis remained essential to fully trust the AI's conclusions.
Data Analysis
Our qualitative analysis involved inductive coding of interview transcripts and observational notes, utilizing thematic, process, and in-vivo coding techniques. We conducted multiple rounds of affinity mapping and theme identification across primary and secondary research data. A signal mapping activity was performed to assess the current landscape of Generative AI, collating diverse viewpoints and understanding the technology's scope. Information was sourced from various online channels, which we classified using the PEST framework.
*To enrich our insights further, we added an "S" for sentiment analysis to the PEST framework, allowing us to gauge the prevailing perceptions of Generative AI—whether optimistic or pessimistic.
Key Themes That Emerged
Collaboration and Integration
A key theme was the integration of Generative AI into design research, with participants envisioning new roles at the intersection of AI and design. Tools like ChatGPT were viewed as valuable starting points, though challenges in establishing information hierarchy and decision-making persisted.
Reliability and Authenticity
We observed a spectrum of responses, with people either over-relying on the technology due to its structured presentation or dismissing it due to inaccuracy and negative past experiences with hallucinations. Concerns about the authenticity of the information emerged continuously as their synthesis methods remained a mystery.
Experimentation and Exploration
Experimentation played a pivotal role in defining how people continued using the tool. We saw an interesting shift occur in the mindset from being an expert to becoming an expert experimenter. Users emphasized the value of iteration, sharing how they “played around” with different approaches to uncover effective strategies.
Points of Intervention
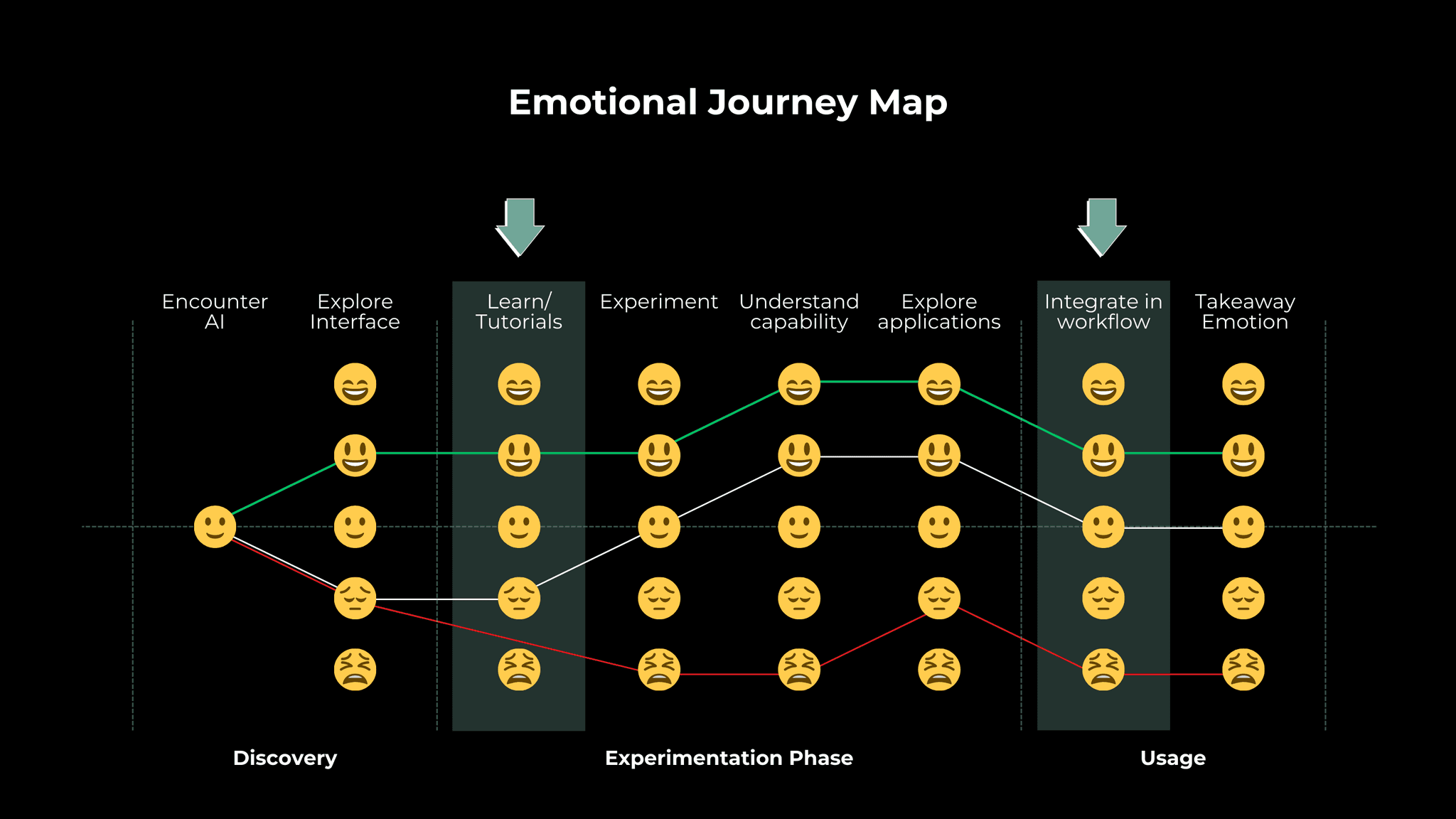
Strategic Pivot
After validating our opportunity statements with professionals we realized that all our statements primarily targeted only those users who were enthusiastic about AI and left behind the ones who were not even ready to adopt them in their practices.
We realized that the main reason behind this was because AI’s findings were often situated out of the communities and hence we combined 2 of the statements and looked at them from the perspective of bias that significantly perpetuates in current AI models and decided to ideate further on that problem statement.
How might we situate Generative AI integration within design research, ensuring its inclusivity and relevance to end-user groups?
Learnings
Solving for Non-Users
The toughest design challenge is often addressing the needs of non-users—those who resist or are excluded from AI integration. Building solutions that are inclusive and meaningful for skeptics requires extra attention and empathy.
Customizing Design Frameworks
A key insight was the importance of tailoring design tools to meet the unique needs of each project, like expanding the 5E framework to 6E to capture emotional and cognitive nuances in the AI adoption journey.
Embracing Shifts
Design research is highly iterative, and the need to pivot can arise unexpectedly at any stage. We made a strategic pivot late in our project, which allowed us to strengthen our focus and ultimately improve our outcomes.